Smart Manufacturing
Topic Co-chairs: Dan Gamota (Jabil), Ranjan Chatterjee (Cimetrix)
Contributing organizations: Cimetrix, Jabil, Palo Alto Networks, SEMI, Kulicke and Soffa Industries
Vision
Definition
The term “Smart Manufacturing” is used when considering the adoption of manufacturing practices across factories, enterprises, and the entire ecosystem, integration of physical and cyber capabilities, and leveraging advanced information for increased flexibility and adaptability of manufacturing.1, 2
The enabling technologies to facilitate this migration include3
leveraging big data infrastructures (along with their data provenance and governance architectures),
adoption across the supply chain network to ensure resiliency and traceability,
leveraging advanced analytics such as techniques based on machine learning and, more generally, artificial intelligence,
improving use of cyber-physical systems (CPS), improving the use of real-time simulation through realizing the “digital twin,” and
reliance on a knowledge network for using subject matter expertise in an increasingly collaborative environment.
At its simplest, smart manufacturing is the integration of (a) physical factory-floor and logistics infrastructure with (b) operational technology (control systems) and (c) information technology (at both the enterprise and the supply chain levels), all while embedding additional intelligence from a variety of tools such as digital twins and machine-learning-based AI. The functional scope is very much that of the industrial automation and control stack shown in Figure 1 below, based mainly on the Purdue Hierarchy.4
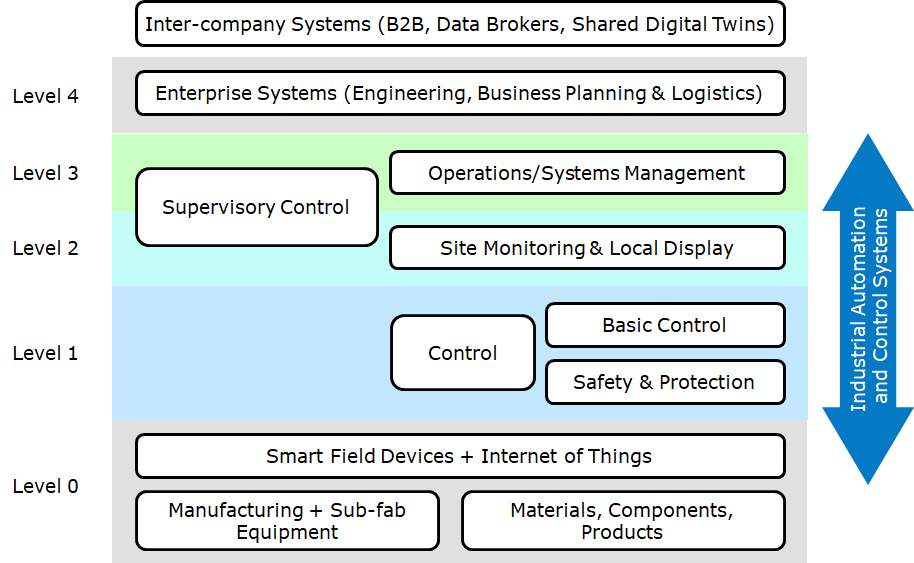
Figure 1: Industrial automation and control stack within the scope of smart manufacturing, based on the Purdue hierarchy.4
Industry context
The vertical segments of the electronic products manufacturing industry―
semiconductor,
outsourced semiconductor assembly and test (OSAT), and
printed circuit board assembly (PCBA),
―are converging and service offerings are being consolidated. OSAT services providers are using equipment and platforms typically found in semiconductor back-end manufacturing and PCBA services providers are installing equipment and developing processes similar to those used by OSAT. This occurrence provides industry members in specific vertical segments an opportunity to capture a greater percentage of the electronics manufacturing industry total profit pool.
As the electronic manufacturing supply chain continues to evolve and experience consolidation, shifts in the traditional flow of materials (e.g., sand to systems) will be facilitated by flexible smart manufacturing solutions.
Readiness indices
Because of the complexity and variability of smart manufacturing installations, a number of frameworks are available to assess the maturity of the overall smart manufacturing infrastructure of an electronics manufacturer. The Smart Industry Readiness Index (SIRI)5 provides a broad readiness index assessment framework for the application of smart manufacturing principles for any manufacturing company. The Semiconductor and Equipment Manufacturing Initiative (SEMI) also has a smart manufacturing readiness assessment framework, the Industry 4.0 Readiness Assessment Model (IRAM)6, developed specifically for electronics manufacturing, with initial focus on semiconductor fabrication with plans to extend to OSAT and PCBA in the near future.
Scope
Topics are organized under four categories: (a) materials flow and conversion, (b) security, (c) data flow architecture and (d) digital building blocks. The main focus is on material flows, manufacturing equipment, factory-floor operational technology (OT) and enterprise information technology (IT) systems. The intersection with product design is considered, but will be expanded upon in later roadmap releases, particularly in the context of digital twins.
Application Drivers
Key drivers for the deployment of smart manufacturing solutions in electronics manufacturing are given in the tables below. These represent the key areas where smart manufacturing create value, both in terms of―
product reliability, cost and speed of production and
managing change and complexity in the supply chain and on the factory floor
―all in the context of rapid changes in product mix and supply chain orchestration.
Table 1. Drivers for the application of smart manufacturing to the electronics manufacturing industry.
Materials Flow and Conversion
Material Handling Systems (MHS) and Automated Materials Handling Systems (AMHS) include the hardware and control systems necessary to transport, store, identify, track, and control the direct and indirect materials necessary for the specific processes performed.
Information on the drivers, needs, and challenges is currently being collected and will be published in 1H24. If you are interested in being notified when this is released please email here.
Security for Smart Manufacturing
Information security will be more challenging with the increase of data shared between manufacturing facilities in an electronics manufacturing vertical (e.g., outsourced semiconductor assembly and test (OSAT)) or between verticals (e.g., between an OSAT and a printed circuit board assembly (PCBA) services provider).
The primary goals of security are to
maintain confidentiality (restriction of access to data and services to specific machines/human users),
maintain integrity (accuracy/completeness of data and correct operation of services),
improve availability (means of measuring a system’s ability to perform a function in a particular time), and
improve hardening to ensure trusted, resilient systems with zero disruption and zero security breaches.
This applies to production facilities, enterprise operations and indeed, the manufactured product.
A detailed view of the needs, gaps, challenges and technology solutions for this topic is given here.
Data Flow Architecture
Data volumes in electronics manufacturing facilities are growing at exponential rates. Pervasive data velocities measured as the data collection rates from equipment increased from less than 1 Hz in the 1990s to 10 Hz in 2020; the roadmap team expects this to be at 100 Hz in a few years with some dedicated sensor data (e.g., the Internet of Things (IoT)) collection rates in excess of 10 KHz.
Data from equipment, maintenance, yield, inventory management, product bill-of-materials (BOM), manufacturing execution system (MES) and enterprise resource planning (ERP) existed for several years; however, analytics tools are evolving to leverage and merge multiple data sources to investigate relationships, detect anomalies, and predict events.
Key elements in emerging data flow architectures are as follows:
Factory information and control systems, or computer integrated manufacturing (CIM), (including computer hardware and software, manufacturing execution systems (MES), decision support systems, factory scheduling, control and diagnostics associated with control of equipment and material handling systems, and process control)
Big data computing (including cloud, edge, and fog computing)
Control systems architectures (including edge, fog, and cloud computing)
Data provenance (detailing data origins, changes to, confidence in, etc.)
A detailed view of the needs, gaps, challenges and technology solutions for this topic is given here.
Digital Building Blocks
Several digital building blocks were identified as enablers of smart manufacturing, as follows: (a) artificial intelligence and machine learning, (b) digital twins, (c) augmented reality/mixed reality, and (d) permissioned blockchains.
Information on the drivers, needs, and challenges is currently being collected and will be published in 1H24. If you are interested in being notified when this is released please email here.
Artificial intelligence and machine learning
Artificial intelligence (AI) in general and machine learning (ML) in particular are key digital building blocks to enable the transition from reactive operations to predictive operations. As predictive operations are adopted there will be significant opportunities for cost reduction, quality improvement, and capacity increases, as well as realized cost benefits from predictive maintenance (PdM), fault prediction, predictive scheduling, and yield prediction.
Digital twins
A digital twin is a connected physics-based simulation model of an in-service asset (see Figure 2). This model emulates the product or equipment behavior in real-time using the same sensor information that the physical asset is experiencing. It mimics and predicts the behavior, enabling operators to fully understand the product life cycle and failure modes of the physical asset in a virtual world.
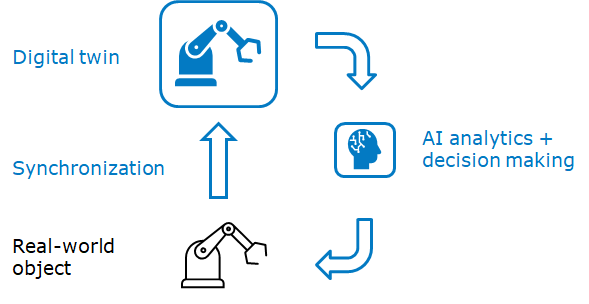
Figure 2: Digital twins in manufacturing
Augmented reality/mixed reality
Hardware devices, such as augmented reality glasses, will provide the individual with a stream of real-time data that aids them in their activities (maintenance, trouble-shooting, etc.) to maximize yield and product quality. These devices will be a key enabler of the smart connected worker―improving their flexibility and productivity in manufacturing facilities.
Permissioned blockchains
In the context of electronics manufacturing, distributed ledger technologies such as blockchain may enable distributed data management platforms where data is shared across a trusted distributed network securely, with traceability, and with embedded business logic. Blockchain technologies have the potential to improve the security and traceability of the entire supply chain, from materials sourcing, to intellectual property (IP) licensing, to eventual production and distribution of integrated products.
Your Feedback
We welcome your comments and suggestions! Here is a link for your input.
References
J. Moyne and J. Iskandar, “Big Data Analytics for Smart Manufacturing: Case Studies in Semiconductor Manufacturing,” Processes Journal, Vol. 5, No. 3, July 2017. Available on-line: http://www.mdpi.com/2227-9717/5/3/39/htm
CESMII, “Accelerating The Democratization Of Smart Manufacturing: 2020 Roadmap Update“, https://www.cesmii.org/wp-content/uploads/2021/05/16124_CES_RoadmapModifications_Digital_rev-final.pdf.
International Roadmap for Devices and Systems, Factory Integration, 2017.
ICIT, The Smart Industry Readiness Index (SIRI), https://incit.org/en/services/siri/, 2023.
SEMI, Industry 4.0 Readiness Assessment Model (IRAM), https://www.semi.org/en/industry-groups/smart-manufacturing/IRAM, 2023.